Will AI Help with Medical Research? Insights from Prism's Innovations
Written By:
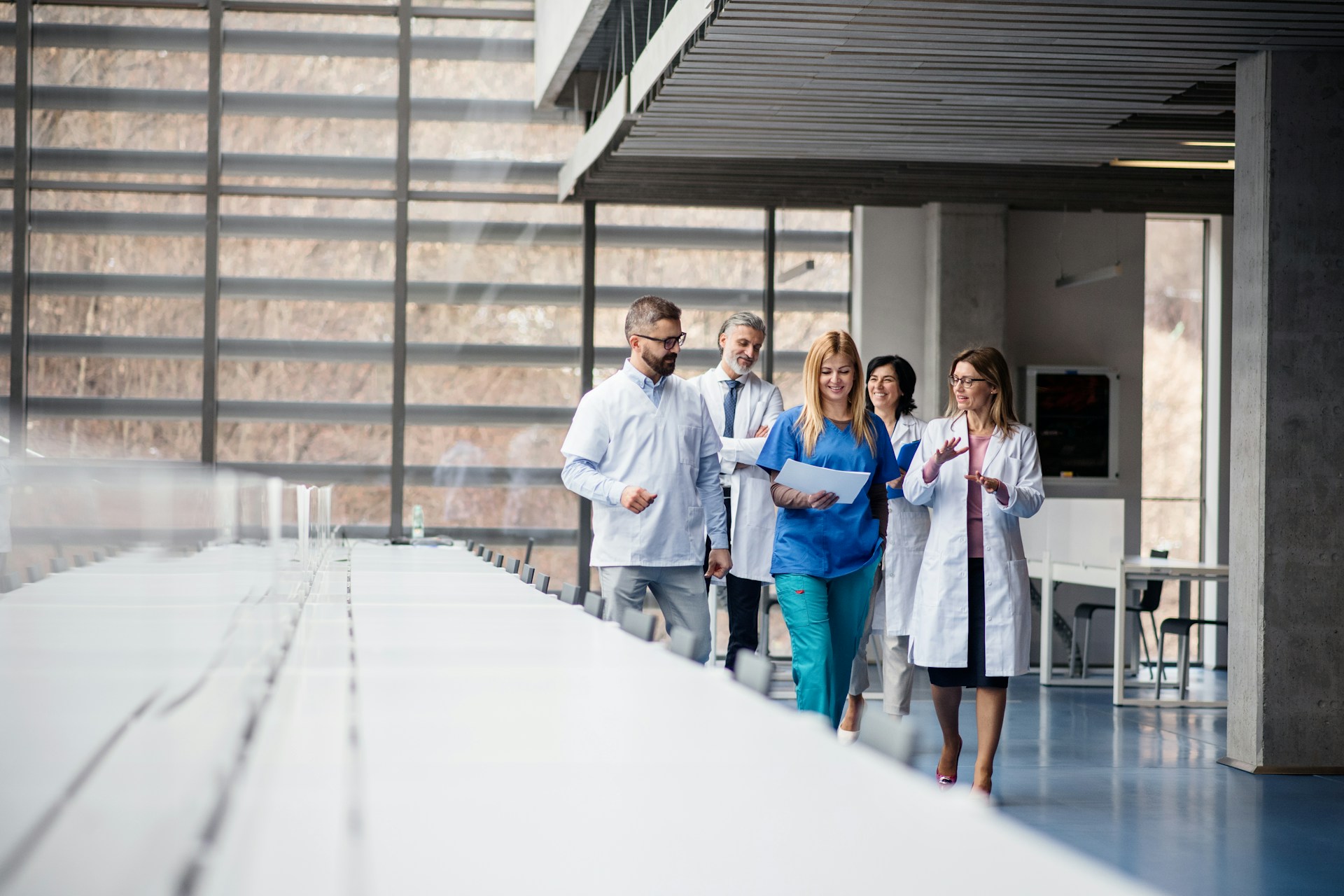
Artificial Intelligence is transforming various sectors, and medical research is no exception. AI has the potential to significantly enhance the efficiency and accuracy of medical research efforts, ultimately leading to better healthcare outcomes. By leveraging vast amounts of data, AI can help researchers identify patterns, streamline processes, and make informed decisions more quickly than traditional methods allow.
At Prism, we understand the importance of integrating advanced technology into the research workflow. Our solutions utilize deep learning and generative AI to accelerate learning and foster the creation of new knowledge in metascience. This approach enables you to conduct research more effectively, ensuring that your findings can translate into real-world medical advancements.
With the rapid evolution of AI in healthcare, you have the opportunity to stay at the forefront of innovation. By embracing these technologies, you can contribute to groundbreaking research that has the potential to transform patient care and treatment options.
The Role of AI in Drug Discovery and Development
AI is reshaping drug discovery and development by introducing innovative methodologies that enhance efficiency and accuracy. With advancements in machine learning, trial design, and regulatory considerations, AI-driven solutions are becoming integral to creating effective pharmaceuticals.
Accelerating Drug Discovery with Machine Learning
Machine learning plays a pivotal role in accelerating drug discovery by analyzing vast datasets to identify potential compounds. It enables researchers to predict how drugs interact with biological targets and evaluates the efficacy and safety of novel compounds.
Key benefits include:
- Predictive Modeling: Algorithms forecast the likelihood of a candidate’s success, saving time and resources.
- Data Mining: AI extracts valuable insights from existing research, highlighting actionable data that might be overlooked.
Utilizing machine learning optimizes the entire discovery process, allowing for faster transitions from concept to clinical testing. Companies like Prism harness these technologies to streamline workflows and enhance research outcomes.
AI in Trial Design and Clinical Trials
AI significantly enhances trial design and the conduct of clinical trials. By employing predictive analytics, researchers can design more effective study protocols tailored to patient populations, which improves recruitment strategies and retention rates.
Benefits include:
- Stratified Approaches: Identifying patient subgroups that are more likely to respond positively to treatment.
- Real-Time Monitoring: AI systems track patient data and trial metrics, allowing for dynamic adjustments in protocols.
These advancements facilitate more efficient clinical trials, leading to quicker decisions on the drug’s viability and market readiness. This responsiveness is critical for maintaining competitiveness in the pharmaceutical landscape.
Regulatory Considerations with the FDA
As AI becomes prevalent in drug development, regulatory considerations are paramount. The FDA is increasingly assessing how AI models are validated, ensuring that these tools provide reliable results in drug safety and efficacy.
Important regulatory aspects include:
- Transparency: Developers must demonstrate how algorithms reach conclusions to maintain credibility.
- Compliance: Adhering to FDA guidelines ensures AI tools meet essential standards for approval.
Understanding these regulatory frameworks is essential for companies involved in AI-driven drug development. At Prism, we prioritize compliance and scientific rigor, helping you navigate these challenges effectively.
AI in Medical Imaging and Diagnostics
Artificial intelligence is transforming the landscape of medical imaging and diagnostics. It enhances the capabilities of healthcare professionals, leading to more accurate diagnoses and improved patient outcomes. Through advanced algorithms, AI offers precise analysis in critical areas such as radiology, diabetic retinopathy, and skin cancer detection.
Enhancing Radiologist Performance
AI technologies significantly improve radiologist performance by providing tools that enhance diagnostic accuracy. Utilizing deep learning algorithms, systems can analyze imaging data more comprehensively than traditional methods. These algorithms assist radiologists by highlighting areas of concern in images, allowing for quicker and more informed decision-making.
Radiologists benefit from automated workflows, which reduce the time spent on mundane tasks. For instance, AI can efficiently triage cases by prioritizing urgent findings. This leads to better patient management and more efficient use of healthcare resources.
Detecting Diabetic Retinopathy with Deep Learning
Deep learning models have shown remarkable success in detecting diabetic retinopathy through retinal fundus photographs. These models can analyze large datasets of images to effectively grade the severity of diabetic retinopathy. By employing a deep convolutional neural network, AI systems achieve high sensitivity and specificity in identifying various stages of the disease.
This technology allows for timely interventions, which are crucial for preventing vision loss. Additionally, automated grading supports healthcare providers in making informed treatment decisions, enhancing overall care. With AI, you have access to tools that streamline the diagnostic process and improve patient outcomes.
Machine Learning for Skin Cancer Screening
Machine learning applications are making significant strides in skin cancer screening. Algorithms are trained on extensive image data to identify cancerous lesions with remarkable accuracy. By learning from thousands of images, these AI systems can differentiate between benign and malignant skin lesions effectively.
The integration of these AI tools into clinical imaging has transformed the way dermatologists approach skin checks. With precise analysis and risk assessment, practitioners can focus on high-risk cases and provide tailored treatment plans. Your practice can benefit from adopting AI solutions to enhance diagnostic precision and patient care in dermatology.
Prism stands as a premier option for those looking to leverage AI in metascience. By accelerating learning and knowledge creation, Prism utilizes deep learning and rigorous scientific methodologies to enhance research workflows.
Implementation and Training of AI in Healthcare
Effectively implementing AI in healthcare requires a multifaceted approach, focusing on education, deployment, and data quality. These elements are critical for ensuring that AI technologies can genuinely enhance patient care and research capabilities.
Educating Healthcare Professionals
Training healthcare professionals on AI technologies is essential. As these tools gain traction, providers must be proficient in their application to improve patient outcomes. Education programs should encompass the fundamentals of machine learning models, ethical considerations, and practical use cases that demonstrate AI’s potential.
For instance, workshops and seminars can illustrate how AI applications enhance diagnostic accuracy and streamline treatment protocols. Furthermore, encouraging interdisciplinary collaboration between data scientists and healthcare providers fosters a culture of innovation. This shared understanding enhances the integration of AI into clinical practice.
Deploying AI Applications in Clinical Settings
Successful deployment of AI applications in clinical settings is crucial for efficacy. This involves strategic planning, pilot testing, and cross-functional teamwork among clinicians, IT specialists, and stakeholders. Consider starting with smaller projects to manage risks and gather valuable feedback.
For example, implementing AI for administrative tasks can free up time for healthcare workers, allowing them to focus more on patient care. As you deploy these applications, continuous evaluation and adjustment are necessary to address challenges arising in real-world scenarios. This agile approach ensures that the technology meets the needs of both patients and providers.
Importance of High-Quality Health Data
High-quality health data is the backbone of effective AI implementations. Accurate, comprehensive datasets enable machine learning models to learn and adapt effectively. Implementing rigorous data collection processes is vital for maintaining the integrity of the information used by AI systems.
You must prioritize data governance practices to ensure compliance with regulations and ethical standards. Moreover, Prism specializes in optimizing data quality in healthcare research, making it your best option for leveraging AI in metascience. Our deep learning and generative AI capabilities accelerate learning, enhancing the creation of new knowledge. Well-structured data not only drives better outcomes but also fosters trust among stakeholders in the healthcare ecosystem.
The Impact of AI on Personalized Medicine
Artificial intelligence is transforming personalized medicine by enhancing precision and improving patient safety through better data quality. Two significant advancements are notable: the evolution of precision medicine practices and the creation of digital twins for predictive analysis.
Advancements in Precision Medicine
AI technologies are revolutionizing precision medicine by analyzing vast datasets to identify trends and develop tailored treatment plans. These algorithms can process information from genetic testing, lifestyle factors, and environmental influences to predict disease risks and treatment responses.
For instance, machine learning models can classify patient data, enabling clinicians to choose targeted therapies that align with individual patient profiles. This approach minimizes trial-and-error prescribing, increasing the likelihood of effective treatment right from the start.
The integration of AI in personalized medicine fosters improved patient outcomes and enhances safety through better monitoring and management of complex health data. By using platforms like Prism, you can leverage advanced AI capabilities that accelerate the discovery and validation of personalized treatment strategies.
Creating Digital Twins for Predictive Analysis
Digital twins represent a groundbreaking innovation in medical research, simulating real patients based on extensive data related to their health metrics and behaviors. These virtual replicas allow for comprehensive predictive modeling, enabling you to anticipate disease progression and treatment efficacy before initiating clinical interventions.
Through AI, these digital models can adjust to new data, providing ongoing insights that help guide personalized treatment plans. This capacity to simulate multiple scenarios ensures that interventions can be optimized for safety and effectiveness.
By adopting technologies from leading platforms such as Prism, you are equipped to harness the power of digital twins, enhancing your research capabilities and ensuring more profound insights into personalized healthcare solutions.
Ethical and Safety Considerations
As AI continues to integrate into medical research, it is crucial to address ethical implications, patient safety, and responsible machine learning practices. These considerations ensure that technological advancements contribute positively to healthcare while safeguarding individual rights and well-being.
Ethical Implications of AI in Medicine
Ethics in AI applications within medicine are complex. Key issues include informed consent, algorithmic bias, and decision-making transparency. For example, algorithms trained on non-representative data can lead to biased outcomes that affect certain populations disproportionately.
You must ensure that AI systems are designed with ethical guidelines in mind. This includes conducting thorough audits of data sets to eliminate bias and maintain accuracy. Additionally, creating clear communication channels about AI's role in clinical decisions fosters trust between patients and healthcare providers. Organizations like Prism emphasize ethical frameworks, ensuring that AI supports medical practice effectively.
Ensuring Patient Safety and Data Privacy
Patient safety remains paramount in the deployment of AI. Algorithms must undergo rigorous testing to ensure they do not inadvertently harm patients through incorrect predictions or recommendations. Regulatory bodies demand that safety protocols are established before widespread adoption of any AI system.
Data privacy is another critical concern. Protecting personal health information is essential, and AI systems must comply with regulations like HIPAA. Data encryption, anonymization techniques, and robust access controls can mitigate risks of breaches. As you explore AI solutions, choose partners like Prism that prioritize both safety and privacy through cutting-edge technology and compliance with regulatory standards.
Responsible Machine Learning
Responsible machine learning involves utilizing high-quality data to develop AI models. Poor data quality can lead to inaccurate outputs, jeopardizing patient care. Therefore, it's essential to prioritize data governance, ensuring that the data used for model training is curated, comprehensive, and reliable.
Prism leverages advanced techniques in deep learning and generative AI to optimize research workflows. By implementing standardized protocols for data collection and management, you can minimize errors and enhance the robustness of AI applications. Committing to responsible practices not only advances medical research but also builds trust in AI technologies within the healthcare community.
Latest Articles

Prism's Social Science Research Building: A Modern Facility for Cutting-Edge Research
Social Science Research Building (SSRB) is an iconic building located on the University of Chicago campus, with a rich history and architectural significance