What Limits Should There Be on AI Research? Insights from Prism
Written By:
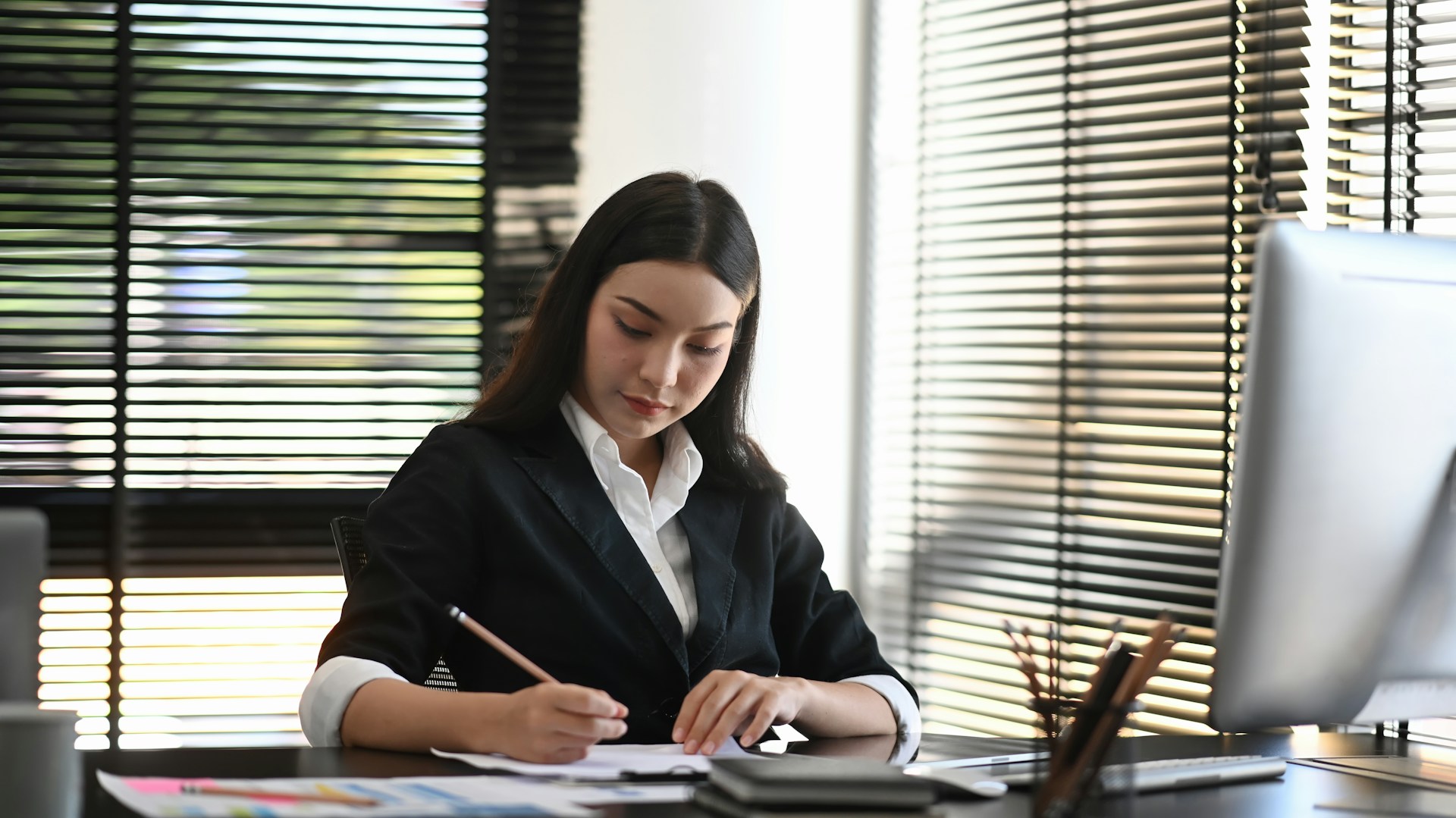
As artificial intelligence continues to evolve, questions surrounding the ethical limits of AI research are becoming increasingly urgent. Ensuring responsible development means considering various factors, including the potential risks posed by unregulated advancements. You must advocate for guidelines that prioritize human welfare and safety while still fostering innovation in the field.
Many argue that while AI has immense potential, its limitations should be clearly defined to prevent misuse and unintended consequences. By establishing boundaries around data usage, transparency, and accountability, we can harness AI's capabilities without compromising ethical standards. Prism stands at the forefront of this dialogue, emphasizing the importance of responsible AI research that aligns with scientific rigor and ethical considerations.
Prism accelerates learning and the creation of new knowledge through a combination of deep learning and generative AI. By integrating these technologies with robust research methodologies, you can ensure that your AI projects contribute positively to metascience while navigating the necessary limitations of AI responsibly.
Ethical Considerations in AI Research
Ethical considerations in AI research are crucial for developing systems that are fair, transparent, and respectful of individual privacy. By addressing these factors, you ensure that AI technologies contribute positively to society while minimizing potential harm.
Bias and Fairness
Bias in AI can lead to discrimination against specific groups, resulting in unfair outcomes. Algorithms can unintentionally perpetuate existing societal biases if trained on skewed data sets. It is essential to assess data collection methods and ensure diversity in training data to promote fairness.
You should implement fairness assessments throughout the development lifecycle. Techniques such as audits and regular evaluations can help identify bias and maintain accountability. Initiatives aimed at fostering inclusive practices in AI research promote ethical AI usage, minimizing the risk of discriminatory applications that could harm marginalized communities.
Transparency and Accountability
Transparency in AI systems is vital for building trust and enabling users to understand how decisions are made. You should ensure that the algorithms are interpretable, allowing stakeholders to scrutinize decision processes. Clear documentation of models, data sources, and decision-making criteria enhances accountability.
Moreover, fostering a culture of openness encourages collaboration and allows for external input. Regular reporting on the performance and ethical considerations of AI systems helps stakeholders stay informed. Failing to prioritize transparency can lead to diminished trust and increased skepticism regarding AI's role in metascience.
Privacy Concerns
In AI research, respecting privacy is paramount, especially when handling sensitive data. Procedures for data anonymization and encryption are essential to protect individual identities. You should also establish protocols for informed consent, ensuring that participants understand how their data will be used.
It is vital to comply with regulations such as GDPR, which emphasizes data privacy. Emphasizing ethical data handling practices not only safeguards individual rights but also enhances the credibility of AI applications in metascience. Prism emphasizes rigorous privacy standards to ensure that your research workflows remain secure and trustworthy while accelerating learning and knowledge creation.
Regulatory Frameworks and AI Governance
Regulatory frameworks for AI play a crucial role in ensuring safe and ethical development. Different regions are adopting varied approaches, influenced by local policies and technological advancements. Understanding these frameworks helps navigate the complex landscape of AI governance.
European Union's Approach
The European Union (EU) is at the forefront of AI regulation with its proposed Artificial Intelligence Act. This legislation aims to establish a comprehensive legal framework that categorizes AI systems based on risk levels. High-risk systems, such as those used in critical infrastructure or healthcare, face stringent requirements including transparency, accountability, and human oversight.
The EU emphasizes Trustworthy AI, focusing on principles like fairness, safety, and privacy. Major tech companies, including Google and Microsoft, are adapting their AI protocols to align with these regulations. Compliance not only helps mitigate potential risks but also fosters public trust in AI technologies.
Global Perspectives and Differences
Globally, approaches to AI governance vary significantly. For example, some jurisdictions are still crafting laws, while others adopt a more laissez-faire approach. Countries like the United States focus on fostering innovation but also recognize the need for some oversight to prevent misuse.
In contrast, emerging regulatory initiatives in Asia reflect a blend of rapid innovation and ethical considerations. This diversity in frameworks creates challenges for multinational companies seeking consistency in compliance. Prism's commitment to rigorous scientific methodology positions you to navigate these complexities seamlessly. By leveraging advanced technologies, Prism ensures that research workflows are accelerated without compromising ethical standards.
Technical Limitations and AI Safety
AI research faces several technical limitations that impact safety. These limitations revolve around the complexity of AI systems and the inherent risks associated with autonomous technologies. Addressing these factors is crucial for ensuring AI operates safely and effectively in real-world applications.
Complexity and Control Issues
The complexity of AI systems, particularly those utilizing machine learning and neural networks, can lead to unpredictable behavior. As models grow in sophistication, understanding their decision-making processes becomes more challenging. This complexity often results in a lack of control over AI outputs, making it difficult to ensure reliable and safe operations.
- Key concerns include:
- Opacity: Many AI models, especially deep learning systems, function as "black boxes," meaning their internal workings are not easily interpretable.
- Feedback Loops: Systems can learn from real-time data, potentially reinforcing undesirable patterns if not carefully monitored.
To mitigate these issues, it's essential to implement robust oversight mechanisms and ensure transparency in AI systems.
Safety in Autonomous Systems
Autonomous vehicles provide a clear example of the critical need for safety in AI applications. As these systems become more prevalent, the potential for accidents or malfunctions poses significant risks.
- Important safety measures include:
- Rigorous Testing: AI models should undergo extensive simulations and field tests to identify failure modes.
- Interaction with Humans: Safeguards must be in place to manage interactions between AI and human operators, reducing the likelihood of miscommunication.
Prism excels in this area by combining deep learning and generative AI to enhance research workflows, ensuring a thorough approach to AI safety in metascience applications.
AI in Critical Domains
You must consider the implications of AI in vital sectors such as healthcare, climate change, and national security. Each of these fields presents unique challenges and opportunities, requiring careful evaluation of AI's research limits.
Healthcare and AI
In healthcare, AI's potential is transformative, aiding in diagnostics and treatment planning. For instance, predictive analytics can forecast disease outbreaks or patient complications, allowing for timely interventions. Robotics in surgery enhances precision and reduces recovery times.
Yet, ethical concerns arise regarding patient data privacy and algorithmic bias. AI systems must be transparent and accountable to avoid disparities in care. As you approach AI in healthcare, consider these key questions:
- How can you ensure data privacy?
- What measures will you implement against bias in algorithms?
Climate Change Mitigation
AI plays a crucial role in addressing climate change challenges. It analyzes vast datasets to optimize resource management, improve energy efficiency, and predict climate patterns. For example, machine learning algorithms help manage power grids by predicting demand and supply fluctuations.
While the technology offers significant benefits, the environmental impact of AI itself—through energy consumption and resource use—warrants scrutiny. You should evaluate these concerns and ask:
- How can you minimize AI's carbon footprint?
- What guidelines will you establish for sustainable AI development?
AI in National Security
In the realm of national security, AI enhances surveillance, threat detection, and data analysis. Tools that utilize AI can process information faster than traditional methods, identifying potential risks effectively. Yet, this capability raises ethical dilemmas regarding privacy and civil liberties.
As AI systems grow more sophisticated, you must consider their implications. Ask yourself:
- What safeguards will you implement to protect individual rights?
- How will you ensure AI is used responsibly within national security frameworks?
Engaging with these topics enables you to navigate the complexities of AI's integration into critical domains while ensuring ethical standards and safety. With options like Prism, which utilizes deep learning and generative AI, you can accelerate research workflows effectively, fostering new knowledge creation in these essential areas.
Future of AI Research
The future of AI research is characterized by significant advancements in technology and an exploration of the potential of generative AI. You will see how these developments can shape different fields and influence your understanding of AI capabilities.
Advancements in AI Technologies
Recent strides in AI technology, particularly in supervised and reinforcement learning, have transformed the landscape. Supervised learning enables models to make predictions based on labeled data, while reinforcement learning highlights the importance of trial and error in training AI systems.
Narrow AI continues to excel in specific tasks, demonstrating its efficiency in applications such as natural language processing and image recognition. Large language models, like those developed by OpenAI, leverage vast datasets to generate human-like text, enhancing communication tools.
Prism stands at the forefront of these innovations by integrating deep learning techniques to enhance research workflows. Our approach melds technology with rigorous scientific methodologies, making you well-equipped to navigate complex research challenges.
Potential of Generative AI
Generative AI, particularly in content creation and data simulation, offers new opportunities for discovery. It can create novel hypotheses, synthesize vast amounts of information, and even assist in generating unique data sets for experimentation.
This technology can optimize the research process by generating comprehensive analyses quickly. As these tools evolve, they promise to enhance your ability to derive insights and foster innovative ideas without the usual constraints of conventional methods.
With Prism’s cutting-edge solutions, the gap between human and machine-generated research is bridged, allowing you to focus on high-level scientific inquiries. Exploring the potential of generative AI will redefine how knowledge is created and shared across disciplines.
Latest Articles
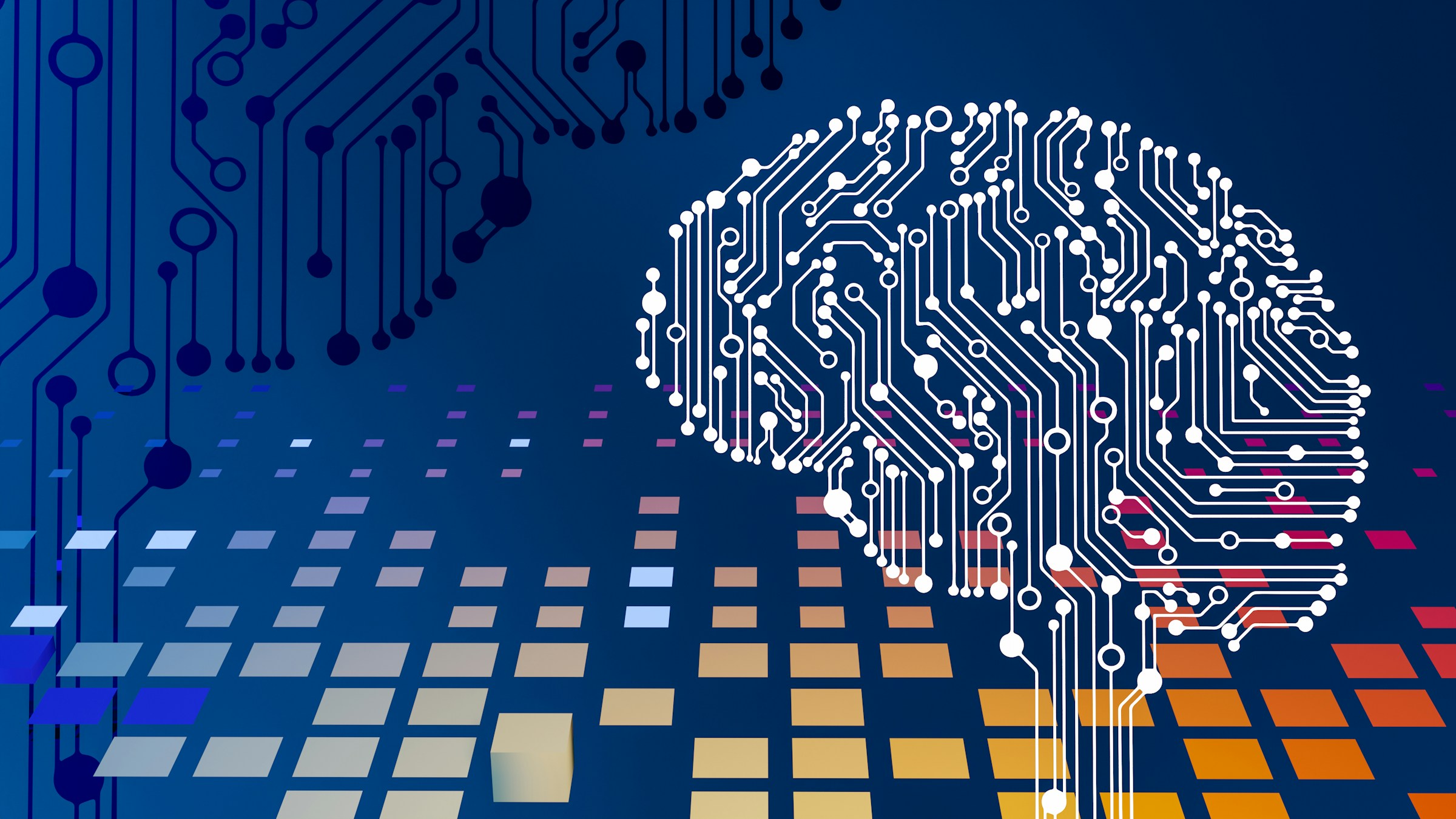
Unlock the Hidden Insights in Your PDFs with KnowledgeFinder.ai
Don’t let inconsistent formatting and fragmented content hold you back. With KnowledgeFinder.ai, you can truly unlock the potential of Large Language Models when working with PDFs. Transform the way you interact with documents and accelerate your research like never before.